News
Autonomous over the field
Robotics in agriculture
Author: Georg Supper
Selected field robots
Dino – Najo Technologies
The company Najo-Technologies was founded in 2012 and has three different robots in its portfolio: Oz, Ted (vineyard) and Dino (vegetables).
Robotti – AgroIntelli
The Robotti field robot is designed for agricultural use in the field. Unlike other robotic solutions, this robot is designed as a tool carrier. It has a three-point linkage, a power take-off shaft, and hydraulic connections. This means that existing attachments up to a working width of 3m can be used. It is driven by a diesel engine. An RTK GPS system is used for navigation in the field. (AgroIntelli 2020).
Thorvald 2 – Saga Robotics
Thorvald 2 is a module-based robot that makes it possible to create very different robot configurations with the same basic modules. The main task ranges from phenotyping in wheat, UV treatment in glasshouse or in foil tunnel depending on the configuration. With this system, it is possible to respond to different applications and agricultural environments by customizing the composition of the robot. The robot is equipped with electric motors and powered by batteries. It is automated using a series of sensors such as GPS and LiDAR (Light Detection and Ranging) sensors and cameras that vary depending on the work environment (indoors vs. outdoors). (Grimstad and From, 2017)
Robot localization
Mobile robots interact with their environment, which they perceive with sensors. Robot programming consequently requires the processing of sensor data. The structure and functionality of sensors have a decisive influence on the design of programs.
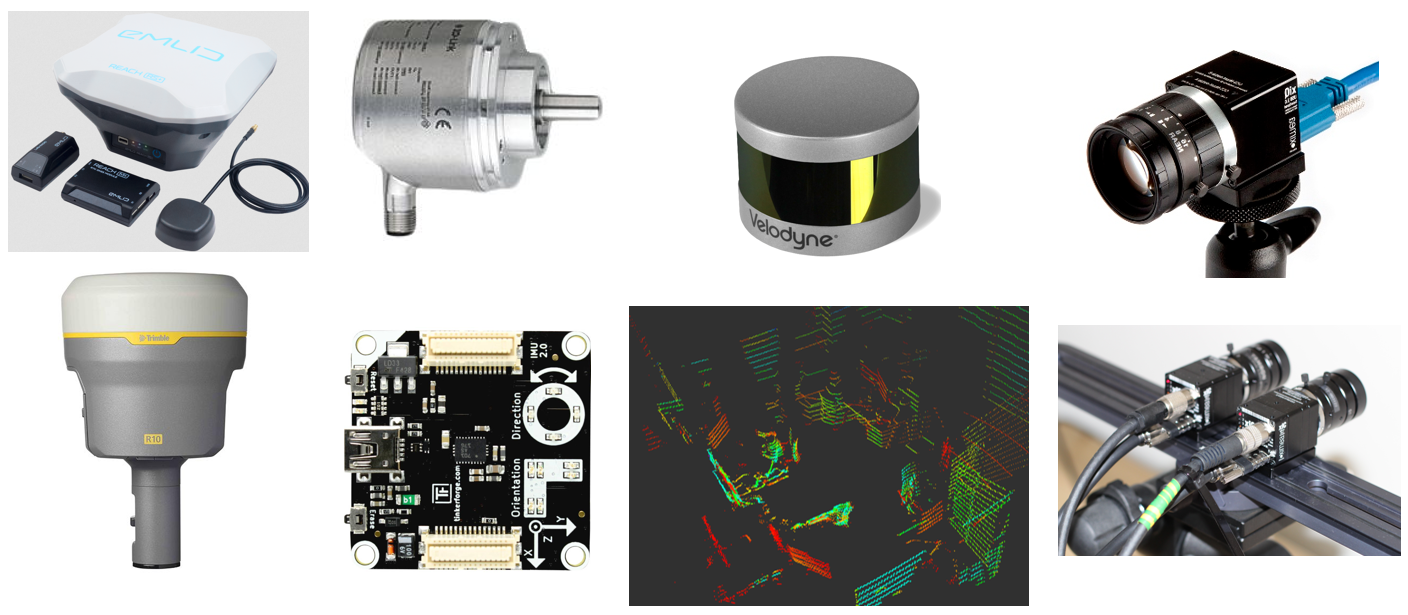
An absolute position is achieved in the field with the help of GPS systems. By using correction services (Real Time Kinematics – RTK) accuracies of 1-2 cm can be achieved. Likewise, 3-D information from laser scanners and stereovision cameras can be used for absolute positioning. Using this data, a map of the environment, and an appropriate algorithm, such as Adaptive Monte Carlo Localization (AMCL), a position estimate can be calculated. This methodology is primarily used for absolute indoor localization.
Supportive or relative positioning is achieved with sensors, such as step encoders, position sensors, and accelerometers. These sensors provide good short-term accuracy, are inexpensive, and allow very high sampling rates. With the information obtained, such as a robot’s travel speed or distance travelled, conclusions about the robot’s position can be drawn using mathematical modelling (e.g., Extended Kalman Filter) of the robot kinematics. Since the basic idea of a relative position measurement is to integrate motion information over time, this leads to an inevitable accumulation of errors. In particular, orientation errors lead to large lateral position errors that increase proportionally with the distance travelled by the robot. Despite these limitations, this is an important component of a robot navigation system.
Machine safety
The safety of people, animals, and objects, but also of the machine itself, is a central requirement in the automation of agriculture. While with industrial robots the work area and thus accessibility can be delimited, this is often not possible in the agricultural sector. This requires a higher level of safety due to a possible more direct physical interaction or a changed working environment. To avoid collisions, the workspace of a mobile robot must be monitored using cameras, laser scanners or other sensors, which places high demands on the robustness of the systems used, especially in changing environments. If there is a risk of collisions, the necessary protective measures must be initiated by the control technology and/or switched to a safe state.
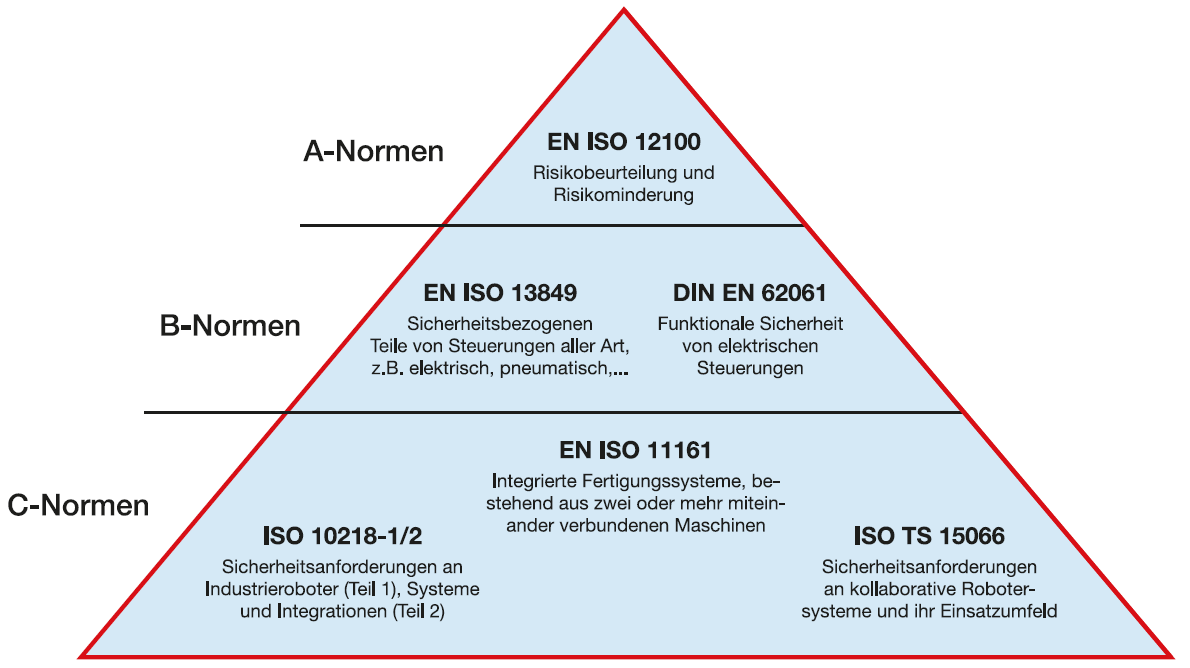
List of references:
AgroIntelli (2020). Robotti. Abgerufen am 21. December 2020, von www.agrointelli.com
Bechar, A. & Vigneault, C. 2016. Agricultural robots for field operations: Concepts and components. Biosystems Engineering, 149, 94-111.
Grimstad, L. & From, P. 2017. The Thorvald II Agricultural Robotic System. 2218-6581, 6.
Hertzberg, J., Lingemann, K., & Nüchter, A. (2012). Mobile Roboter: Eine Einführung aus Sicht der Informatik. Springer-Verlag.
Jacobs, T. 2013: Validierung der funktionalen Sicherheit bei der mobilen Manipulation mit Servicerobotern – Anwenderleitfaden. Frauenhofer- Institut für Produktionstechnik und Automatisierung IPA.
Naio Technology (2020). Dino – Vegetable robot. Abgerufen am 21. December 2020, von /www.naio-technologies.com
SPARC. 2015. Robotics 2020 Multi-Annual Roadmap—For Robotics in Europe [Online]. http://sparc-robotics.eu/wp-content/uploads/2014/05/H2020-Robotics-Multi-Annual-Roadmap-ICT-2016.pdf.
SCHWICH, S., STASEWITSCH, I., FRICKE, M. & SCHATTENBERG, J. 2019. Übersicht zur Feld-Robotik in der Landtechnik. Jahrbuch Agrartechnik 2018, Band